Geographic Privacy-aware Knowledge Discovery and Delivery
Authors
- Fosca Giannotti (CNR Pisa, Italy)
- Dino Pedreschi (University of Pisa, Italy)
- Yannis Theodoridis (University of Piraeus, Greence)
Abstract
A flood of data pertinent to moving objects is available today, and will be more in the near future, particularly due to the automated collection of privacy-sensitive telecom data from mobile phones and other location-aware devices. Such wealth of data, referenced both in space and time, may enable novel classes of applications of high societal and economic impact, provided that the discovery of consumable and concise knowledge out of these raw data is made possible. Recent research activities have developed theory, techniques and systems for geographic knowledge discovery and delivery, some of them based on privacy-preserving methods for extracting knowledge from large amounts of raw data referenced in space and time. All these efforts aim at devising knowledge discovery and analysis methods for trajectories of moving objects.
The fundamental hypothesis is that it is possible, in principle, to aid citizens in their mobile activities by analysing the traces of their past activities by means of data mining techniques. For instance, behavioural patterns derived from mobile trajectories may allow inducing traffic flow information, capable to help people travel efficiently, to help public administrations in traffic-related decision making for sustainable mobility and security management, as well as to help mobile operators in optimising bandwidth and power allocation on the network. On the other hand, it is clear that the use of personal sensitive data arouses concerns about citizens' privacy rights.
In this tutorial, we establish a framework for the challenges and the mining solutions for the geographic information collected by Moving Object Database (MOD) engines. We first discuss the challenges of collecting mobility data, and elaborate on the impact of trajectory data analysis in several modern applications. We then discuss methodologies and techniques to collect raw data, reconstruct trajectory information, and efficiently store it in MODs. We continue with an overview of knowledge discovery approaches for movement data. Finally, we propose a research agenda and identify areas where interdisciplinary studies are needed.
About the Authors
Yannis Theodoridis (University of Piraeus, Greence)
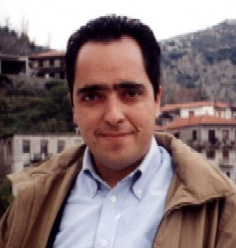
Yannis Theodoridis is Assoc. Professor with the Department of Informatics, University of Piraeus in Greece. He received his Diploma and PhD degrees in computer engineering from the National Technical University of Athens in 1990 and 1996, respectively. Since 2002, he is in the faculty of Informatics, University of Piraeus in Greece, currently directing the Information Systems Laboratory (InfoLab). His research interests include knowledge discovery and data mining (KDD), spatial / spatiotemporal databases and geographical information systems (GIS). He was scientist in charge and coordinator of two European FP6 projects, namely PANDA (IST-2001-33058 FET Working Group; 2001-04) and CODMINE (IST-2001-39151 FET Assessment Project; 2002-03), on pattern-based management and privacy-preserving data mining, whereas he is currently involved in GeoPKDD Project (IST STREP Project, 2005-05) on moving objects trajectory warehousing and mining aspects. Yannis Theodoridis has presented tutorials on spatial databases (ADBIS'99) and mobile location solutions (ADBIS'03).